Robin Schmucker
rschmuck[at]cs.cmu.edu
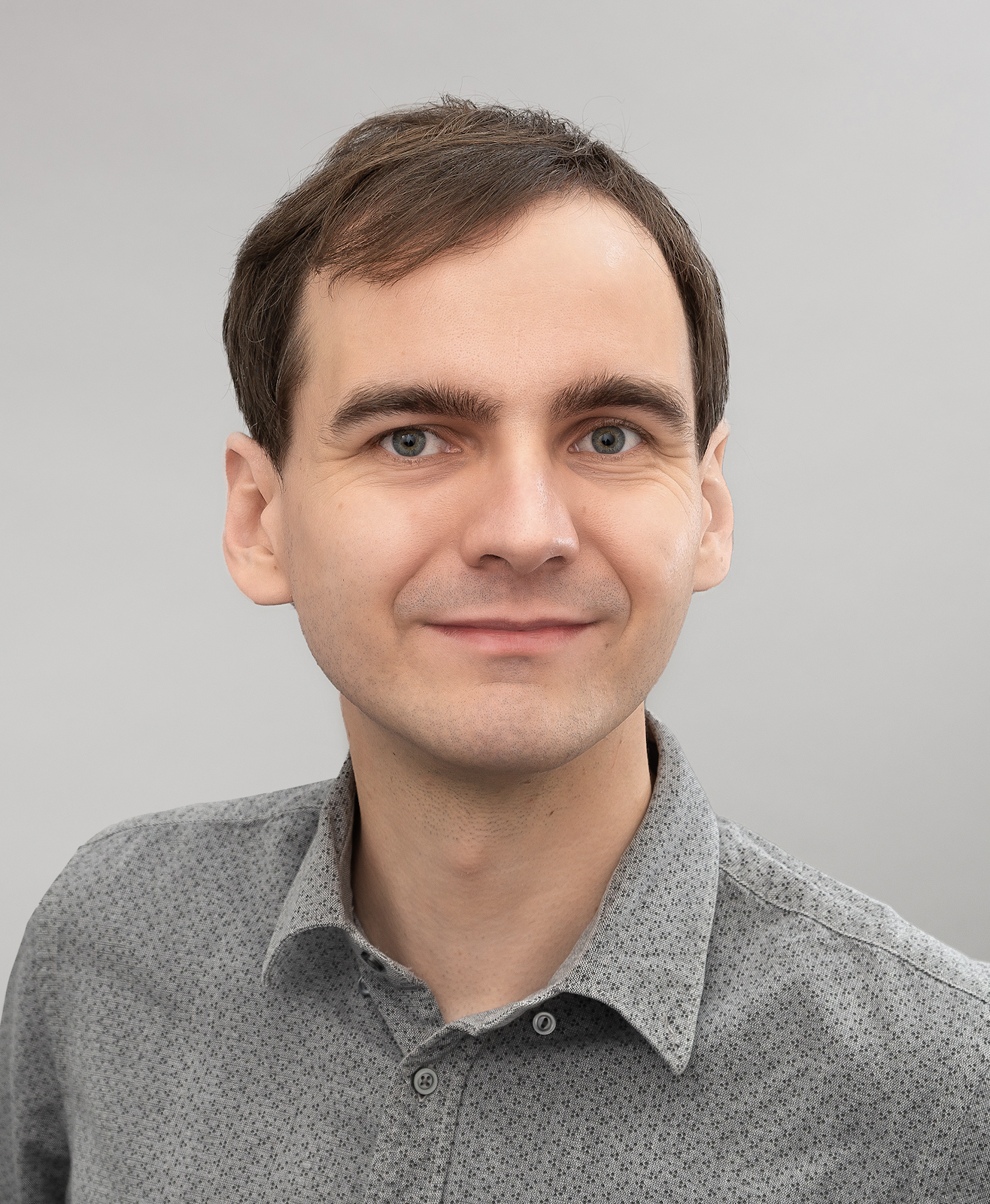
8227 Gates Hillman Center
4902 Forbes Ave
Pittsburgh, PA 15213
Welcome! I am a postdoctoral researcher with joint affiliations at Carnegie Mellon University, under the guidance of Prof. Tom Mitchell, and the University of California, Berkeley, where I collaborate with Prof. Zachary Pardos.
My research focuses on machine learning and human-AI interaction, particularly in the context of large-scale online education. I develop data-driven algorithms and systems to promote more effective, scalable, and equitable learning experiences. Some questions I am actively pursuing:
-
What can we learn about student knowledge acquistion using modern machine learning and robust statistical methods? [1, 2, 3, 4]
-
How can reinforcement learning help us understand the effects of instructional materials and refine the abilities of learning systems? [1, 2]
-
How can generative-AI facilitate structured conversational learning activities and foster new types of content authoring tools? [1, 2]
We are grateful to collaborate with the CK-12 Foundation, where our algorithms for student knowledge modeling and content selection benefit millions of learners worldwide.
Previously, I completed my PhD in the Machine Learning Department at CMU. I studied computer science at KIT in Germany. I was a research assistant at TECO with Prof. Michael Beigl. Supported by the CLICS fellowship, I worked on human-robot interaction advised by Prof. Manuela Veloso. In the industry, I was a research intern at AWS where I designed new algorithms for multi-objective hyperparameter optimization and contributed to AutoGluon.
Research opportunities: I am happy to collaborate, discuss research and answer questions about CMU’s academic programs. If you are interested, please feel free to send me an email.
I am on the job market for positions in machine learning, human-AI interaction, education and related disciplines.
news
Dec 18, 2024 | Our paper on AI Mentors for Student Projects got accepted as a spotlight at AAAI-iRaise. |
---|---|
Nov 05, 2024 | Honored to give talks about LLM-based conversational tutoring at UPenn and WPI. |
Oct 03, 2024 | I successfully defended my PhD thesis on Sequence-Modeling for Assessments and Interventions in Intelligent Tutoring Systems. I am deeply thankful to the many friends and collaborators who contributed to my dissertation, both directly and indirectly. |
Jul 28, 2024 | Our project Artificial Mentors for Student-Driven Projects won a Tools Competiton Catalyst award. We develop LLM-based technologies to support project-based learning activities. |
Jul 06, 2024 | Looking forward to two weeks of insightful discussions and presentations at AIED and L@S. We are honored to present several of our recent works [1,2,3]. |